Integration of physics and machine learning models to further improve solar forecast
Submitter
Liu, Yangang — Brookhaven National Laboratory
Area of Research
Radiation Processes
Journal Reference
Liu W, Y Liu, T Zhang, Y Han, X Zhou, Y Xie, and S Yoo. 2022. "Use of physics to improve solar forecast: Part II, machine learning and model interpretability." 244, 10.1016/j.solener.2022.08.040.
Science
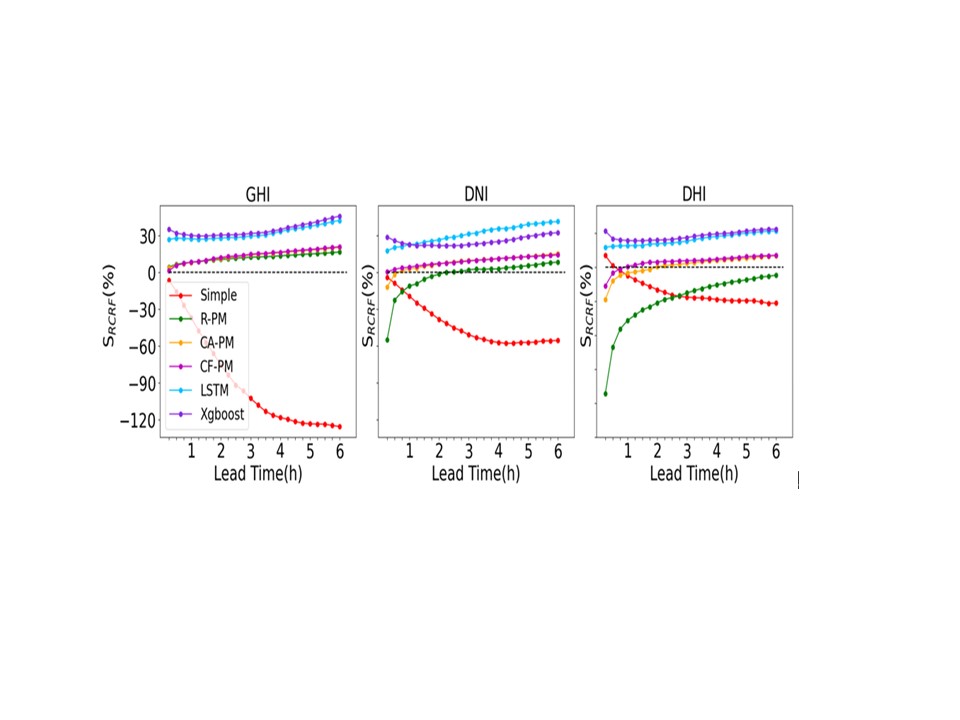
Figure 1. This figure compares the forecast skill of different models relative to one of our physics-informed persistence models (RCRF-PM) presented in Part I (Solar Energy 215: 252-265, https://doi.org/10.1016/j.solener.2020.12.045). The left, middle, and right panels are for Global Horizontal Irradiance (GHI), Direct Normal Irradiance (DNI), and Diffuse Horizontal Irradiance (DHI), respectively. Please see the paper and Part I for details on the models and acronyms. From journal.
Long-term radiative measurements collected at the U.S. Department of Energy's Atmospheric Radiation Measurement (ARM) user facility Southern Great Plains (SGP) observatory are used to develop physics-infomed machine learning models to further improve simultaneous forecasting of global horizontal irradiance (GHI), direct normal irradiance (DNI), and diffuse horizontal irradiance (DHI).
Impact
This study demonstrates the potential of incorporating simple physical relationships underlying cloud-radiation interactions into data-driven machine learning models to improve solar irradiance forecast and model interpretibility.
Summary
Observation-based statistical models have been widely used in forecasting solar energy; however, existing models often lack a clear relation to physics and are limited largely to global horizontal irradiance (GHI) forecasts over
relatively short time horizons (<1 h). As the second paper of our sequence of incorporating physics into observation-based models to increase forecast time horizons and accuracy of forecasting not only GHI but also direct normal irradiance (DNI) and diffuse horizontal irradiance (DHI), this study incorporates simple physical-cloud-radiation relationships to machine learning models to further improve solar forecasts. The long-term, broad-band radiation measurements at ARM’s SGP Central Facility are used to train and evaluate the performance of the machine learning models. The results show that the new physics-informed forecast models generally outperform the commonly used simple and smart persistence models, and our new physics-informed persistence models, presented in Part I. Integration of simple cloud-radiation physics and machine learning models, enhance model interpretibility as well.
Keep up with the Atmospheric Observer
Updates on ARM news, events, and opportunities delivered to your inbox
ARM User Profile
ARM welcomes users from all institutions and nations. A free ARM user account is needed to access ARM data.