Machine learning techniques speed up aircraft aerosol mass spectrometer Analyses
Submitter
Shrivastava, Manishkumar — Pacific Northwest National Laboratory
Area of Research
Aerosol Properties
Journal Reference
Pande P, M Shrivastava, J Shilling, A Zelenyuk, Q Zhang, Q Chen, N Ng, Y Zhang, M Takeuchi, T Nah, Q Rasool, Y Zhang, B Zhao, and Y Liu. 2022. "Novel Application of Machine Learning Techniques for Rapid Source Apportionment of Aerosol Mass Spectrometer Datasets." 6(4), 10.1021/acsearthspacechem.1c00344.
Science
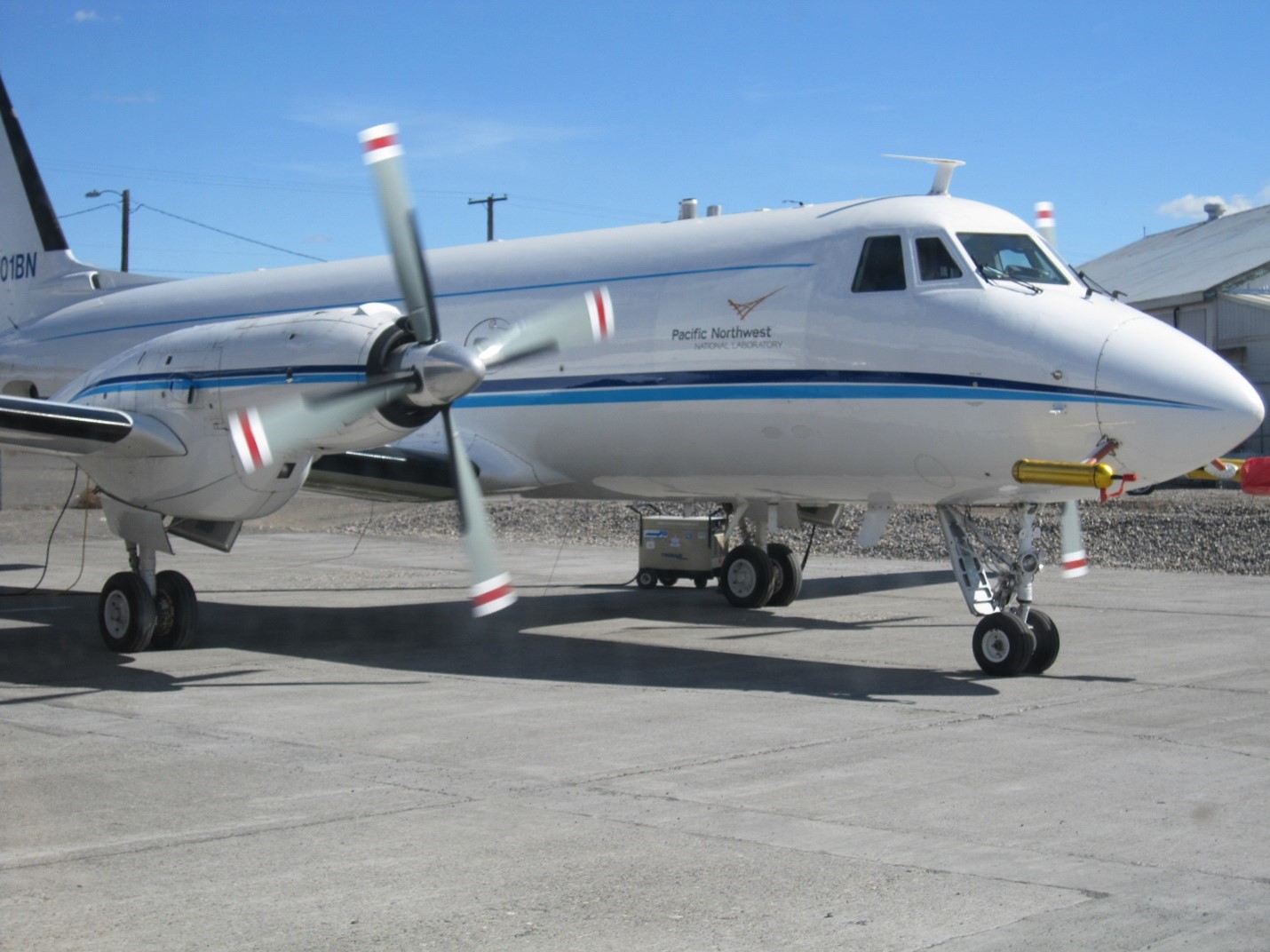
Figure 1. Using machine learning, researchers can quickly identify the composition of organic aerosols based on mass spectrometer data. (Image courtesy of John Shilling|Pacific Northwest National Laboratory)
Human and natural emissions contribute to the formation of fine particles in the atmosphere, including organic aerosols (OA). Aerosol mass spectrometers (AMS) are widely used to measure the composition of organic aerosols. Commonly, researchers use the positive matrix factorization (PMF) technique to derive the mass fractional contributions of different sources of OAs from AMS data. However, PMF analyses need substantial user judgement to relate PMF factors to sources and are especially challenging for aircraft measurements. Researchers developed a new capability that applies machine learning techniques to rapidly apportion OA mass spectra to predefined sources. It can be applied to single mass spectrum rather than the full AMS data set. This approach can be applied online as AMS data are being collected, without substantial user judgements.
Impact
This work presents a novel application of two-step supervised machine learning techniques to AMS data analyses. Thus far, PMF has been the de facto approach for AMS data analyses. Once trained, this machine learning approach can be used to rapidly determine OA sources for both aircraft- and ground-based AMS measurements to complement time-consuming PMF analyses. The approach has potential applications for a variety of past and upcoming field measurements since it can yield results in seconds and analyze single samples.
Summary
New research applies supervised machine learning approaches—sparse multinomial logistic regression and ensemble regression—to classify AMS data and then apportion the OA data to sources. The classifier was trained to identify eight OA types using 60 well-characterized reference spectra. These include four laboratory-derived secondary organic aerosol (SOA) spectra as well as PMF deconvolved spectra for three primary organic aerosol (POA) types and a more oxidized oxygenated OA type. Next, an ensemble regression model was trained on an artificially generated data set consisting of mixtures of different OA types. This allows the model to predict fractional mass abundances of various OA species from classification probabilities obtained from the classifier trained on the reference spectra. Ultimately, the proposed approach was applied for source apportionment of aircraft-based AMS measurements during the Holistic Interactions of Shallow Clouds, Aerosols and Land Ecosystems (HI-SCALE) field campaign. On two representative days (May 6th and 18th, 2016), the algorithm determined that ∼50−60% of OA by mass was more oxidized oxygenated OA, representing a highly aged organic aerosol mixture from different sources. On both days, the method determined that biomass burning OA contributed less than 10% to OA by mass. The proposed approach is capable of rapidly analyzing AMS data in real time, making it suitable for applications where rapid source apportionment of AMS OA spectra is desirable.
Keep up with the Atmospheric Observer
Updates on ARM news, events, and opportunities delivered to your inbox
ARM User Profile
ARM welcomes users from all institutions and nations. A free ARM user account is needed to access ARM data.